Figure C5h. Project Management, Engineering, and Science in a Data Pool (DP)
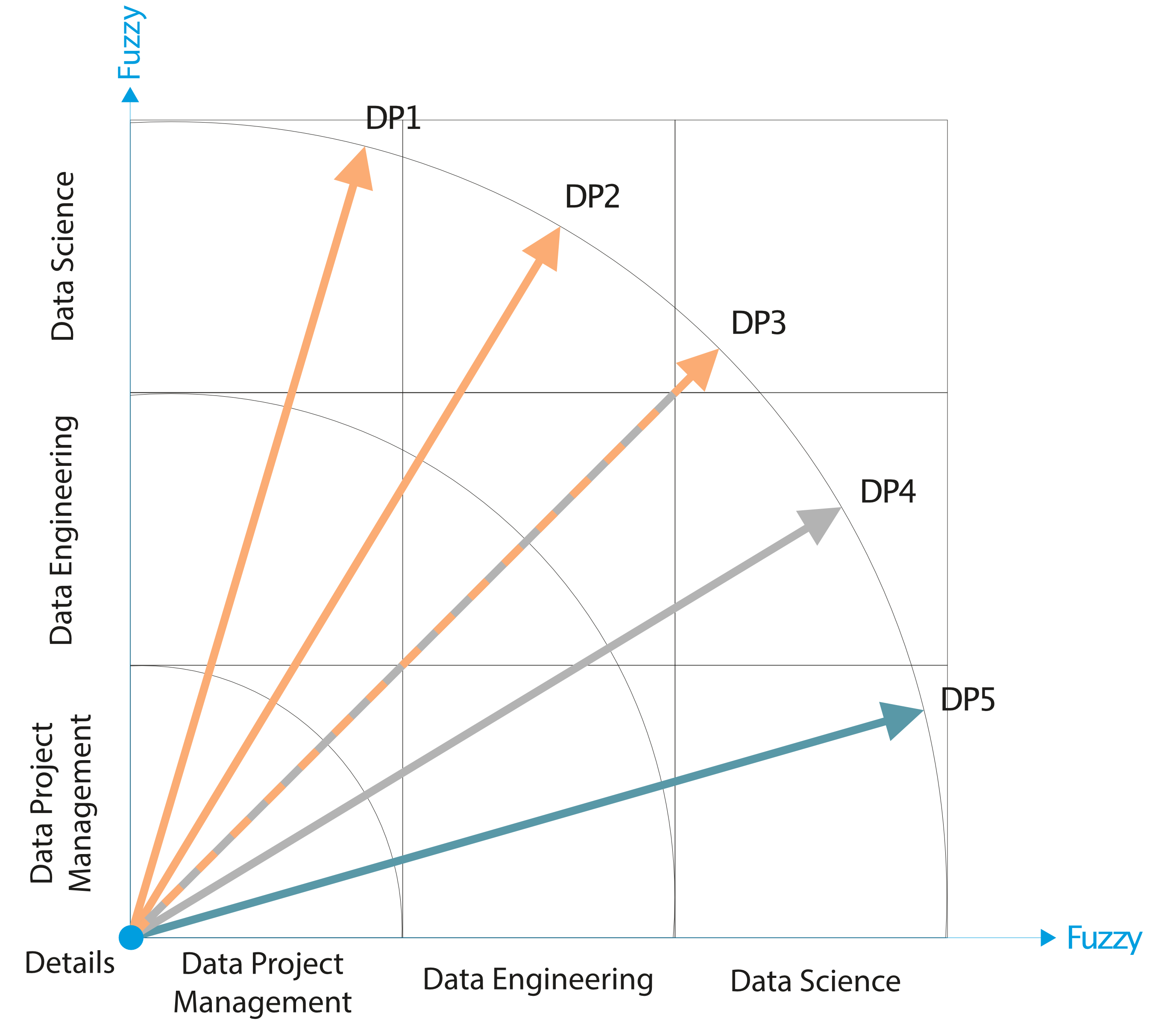
DPM (Data Project Management)
DPM is a project management plan. Project management includes planning, initiating, executing, monitoring, and closing a project on an elementary level of data. Many different project management methodologies and techniques exist, including traditional and still more and more digitized ones (e.g., Easy Software Ltd.).
Further general details about DPM are presented through other segments of this chapter. In the following chapters (mostly D and E), the DPM will have a more specific description of the content in a link to the SPC Utility and SPC Drivers.
DE (Data Engineering)
DE is a matter of data science that focuses on practical data collection and analysis applications. DE generates data, and data scientists must answer questions using large sets of information. DE operates mechanisms for collecting and validating that information.
For the needs of this webbook, the processes of the DE are presented as (details) base of the Data Project Management (DPM) in an integrated environment of a Data Lake (DL) that is broken down on a chain of inner Data Pools (DPs).
On the other hand, DE activities have links to activities of DS (Data Science).
In this direction, the DE is giving technological support to the activities of Data Science (DS).
The global synergy of DE with the Data Project Management (DPM) on one side and with DS on the other enables and disseminates the "culture" growth of project management worldwide (see comments to the new project paradigm in this webbook).
DE is the complex task of making raw data available to data scientists and groups within an organization. Data engineering includes several data science specializations.
They know how to cooperate with scientists (e.g., in Artificial Intelligence and Machine Learning tasks) to build and maintain database systems, master programming languages, and prepare and implement ICT projects for organizations and projects. It is an area of cognitive science.
Data engineering helps make data more valuable and accessible for consumers of data. Engineering sources, transform and analyze data from each system.
For example, data stored in a relational database can operate as tables, like a Microsoft Excel spreadsheet (Microsoft Excel now supports 1,048,576 rows and 16,384 columns, about 17 billion cell’s).
Data size and their growth characteristics cover big and small size data. Big data involves more significant quantities of information while small data is, not surprisingly, smaller. We can say that big data helps describe massive chunks of unstructured information. On the other hand, small data involves more precise, bite-sized metrics.
DS (Data Science)
DS covers methods, processes, algorithms, and systems to extract information from structured and unstructured data and apply knowledge and actionable insights from data across a broad range of application domains.
DS is the highest (general) level of data operations in a Data Lake (DL). Data Science (DS) is opening the door and supports the understanding and growth of knowledge about the algorithm of living organisms.
Medicine and its scientific disciplines shape future applications of DS. However, many inspirations come from the Data Science (DS) algorithms for Data Project Management (DPM) applications we can create today (some ideas and examples are present in this web book).
Data science is an interdisciplinary field that uses scientific methods, processes, algorithms, and systems to extract knowledge and insights of structured and unstructured data across a broad range of application domains.
Data capture is the process of collecting data from high-end technologies to low-end paper instruments still frequently used.
We have known that data is the "blood" of the digital transformation system, and data understanding in scaling, setting up algorithms, and preparing their structure for practical tasks of the scientific and engineering data professional disciplines.
Scientific data are defined as information collected using specific methods for a particular purpose of study or analysis.
For example, data collected for a laboratory experiment (temperature, talk), economic data (gross domestic product), data of organizations (added value), project data (efficiency, economy, effectiveness).
What is the difference between data and big data? Any definition is circular, as "Big" data is still data, of course. It is a set of qualitative or quantitative variables – it can be structured or unstructured, machine-readable, or not, digital or analog, personal or not.
Therefore, Big Data is not just "more" data. Small data is data that is 'small' enough for human comprehension. Data in a volume and format makes it accessible, informative, and actionable.
We can find this definition on the internet: "big data" is about machines, and "small data" is about people. In a nutshell, simple enough data for human understanding in such a volume and structure makes it accessible, concise, and workable. We know it as small data around us every day.
Figure C5h presents Data Pools (DPs) as vectors in a matrix of Data Project Management, Data Engineering, and Data Science segments. In other words, these are significant quantities of data flows in the project's life cycle (portfolio).
The directions of the vectors indicate the development and position of the function of individual DPs formed under the influence of essential project tools (Project Management, Data Engineering, and Data Science). The diagram aims to show how to monitor and influence the synergy of the impacts of all tools available to the project life cycle (project portfolio).
Figure C5i presents a similar approach to data content and structure. The difference is that data sets, strings, and their evaluation, as they are forming, are confronted with opportunities to transfer the results of science, research, and other good practice into individual projects.
The aim is the same, to indicate the way for the use of analyzes and offers of applications of science and research into files and strings of data in an individual DP.
Each DP represents a different environment in which, under certain conditions, it is feasible to standardize content similarities to data compliance and automate them. The application of machines in project preparation and implementation processes is more detailed in Chapter D.
Figure C5i indicates the entry of Artificial Intelligence (AI) into the processes of Data Pools through six vectors (Programming, Pro; Statistic & Probability, S&P; Machine Learning, MT; Big Data operations, BD; Data Analysis, DA; and Internal Research operations, IR). Proposed vectors form the framework of new technology, e.g., Blockchain and Smart Contract.
The picture aims to learn to perceive and use tools concerning the dynamically growing knowledge in the fields of IA.
At the same time, the Figure provokes questions on how to compare the uneven extent of absorption capacity (with a digital inclusion) in territorial units, which, whether they will want to, will be permanently affected by the effects of AI.
Can responses to such questions be constructive and emotionless? Without a fear that this is a topic that local project stakeholders will not understand. The answer is yes.
Project stakeholders and their final beneficiaries will realize it because it is about the structures of the processes they know (e.g., speed up the building processes, control the quality of execution, prevent corruption, eliminate theft, keep the secrecy of what is to be secret).
For example, we can imagine a project about extracting drinking water (surface and groundwater), its transport (transmissions), and its distribution to end consumers (see more in Chapter 9 and Chapters D, E, and F).